Count & speak number of buttons
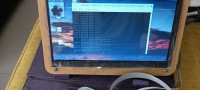
Count object using edgeimpulse is very simple and python SDK can be used further to tailor made the project output. The device used is Raspberry Pi with camera. It counts objects and then speaks out through the speaker connected to the Raspberry Pi computer.
Count buttons by edge impulse
Prelude : Classify of object is easily possible by edge impulse models. You can easily identify between a man & animals, between bi-cycle & other types of cars and so on. In the same coin you can easily count one type of object among other types of objects. This project at the beginning was aimed to install on MCU level – ESP32, Arduino Nicla vision etc. And that’s why it was made for very small area of counting [120 pixel x 120 pixel ] with relatively small size button as object of interest but finally it transpired that even for the smallest area too the MCUs are no match at all. The model file itself is about 8MB long! Therefore, the project was finally installed in a Raspberry Pi computer where it works easily.
Edge Impulse: First open an account in edgeimpulse.com which requires an email id. Collect similar types of buttons handful numbers. If you open the site from a Raspberry Pi computer, using the camera of the raspberry pi computer [either USB connected or cam port connected] you can collect images of buttons from several angles [which is required while the model is deployed in real working field]. However, edge impulse has provisions to connect your cell phone or laptop etc. to connect to for input device which is also more convenient for data acquisition in edge impulse project.
The project : The edge impulse project is broadly divided in the following steps –
1. Data acquisition – this could be image, sound, temperatures, distances etc. Part of these data are separated as test data while all other data are used as train data.2. Impulse design – this is sub divided into Create Impulse – sub divided by1. Input parameters – image [ width, heigh], sound [sound parameter]2. Processing block - How to process the input data3. Learning block - [object data of this model]
3. Image processing - Generate Feature of the collected images
5. Object detection – Select your Neural Network model and train the model.
In the final part – the object detection part needs your expertise or I would rather call it – trial and error effort so that the accuracy of the model becomes 85% or above. There are quite a handful models which you can try and see the accuracy level of the model. Anything above 90% is great but certainly it should not be 100% accurate! If it is so then you have something wrong in your data – could be very less data or insufficient features are there. Recheck & retry again for that case! For this project the accuracy was 98.6%. Certainly our number of data [about 40] was less. However, for a starter project this is pretty good! See the picture below.
Model Testing: You can test your model on the test data first. See how it works out there and then point your device to the real life data and see how it works! On the browser page look at this feature –
In the dashboard of the edge impulse opening page this feature is available. You can scan the image on your mobile and then run the model there or you can straight away run it in the browser. Point the camera to the buttons and see whether it can able to count them or not!
Raspberry Pi deployment : For running the model on Raspberry Pi computer you have to download the *.eim file on raspberry pi computer. But unlike for other hardware [Arduino, Nicla Vision, ESP32 where you can download directly] in case of Raspberry Pi you have to install edge impulse on the Raspberry Pi computer first. From inside that edge-impulse-daemon software you have to download this file. But don’t worry, edgeimpulse has devoted a full page for installing edgeimpulse on Raspberry Pi. Look at here – it’s pretty easy.
https://docs.edgeimpulse.com/docs/development-platforms/officially-supported-cpu-gpu-targets/raspberry-pi-4
OK, so you have installed edgempulse on the Raspberry Pi computer now. Now Run
edge-impulse-linux-runner [Remember to keep the Internet on for Raspberry Pi now]
on a Raspberry Pi computer terminal and see it connects to your edge impulse page [run edge-impulse-linux-runner --clean to switch projects]. This command will automatically compile and download the AI model of your project and then start running on your Raspberry Pi computer. [see command] Show the buttons to the camera connected to your Raspberry Pi and it would count them.
Deploy model in python: OK so far so good. In the above deployment, it would work as it is intended in the edge impulse model. To make it work for your special purpose , say raise an audio alarm / light an LED when the count reaches +2 you have to find some other means! Here comes python3 to help you out. Linux-sdk-python needs to be installed now in your Raspberry Pi computer.
Edge impulse SDK [Short form for Software Development Kit] is available for many models – Python, Node.js, C++ etc. The below link is for taking you to the page for SDK python page.
https://docs.edgeimpulse.com/docs/tools/edge-impulse-for-linux/linux-python-sdk
Once the Linux-SDK-Python is installed then go to the linux-sdk-python/examples/image directory and run the python file for image identification. Don’t get confused. In the example directory there are three sub directories – one each for audio data, image data and custom data. In the image directory the video classification file is also available for video input data. The custom directory is for customizing other kinds of data [ For experts area only!].
$> python3 classify-image.py /home/bera/downloads/model.eim
That’s how you have to load the python file with the downloaded model.eim file. The program will automatically find the camera module [USB connected or Cam-Port connected] and will start running! On the top left corner a small 120x120 camera window will open and the identified buttons will be market with small spot! The numbers identified will be shown on the terminal. Please ensure sufficient light is available and the camera is properly focused for the buttons. This is particularly important for cheap cameras. That’s why if you run the model on your smart phone it produces far superior images and counts far more quickly. Nonetheless ensure proper light and focus, it will also produce better result.
Customize your model: Please have a look into the classify-image.py file and it’s a not-a-simple python file which can be tailor made with little understanding. In this python file I’ve added espeak module such that the moment it finds button(s) ,it speaks out the number of button(s) it finds.
Lets see part of the python file to work around:-
#!/usr/bin/env pythonimport device_patches # Device Specific patches – taken care by the softwareimport cv2 #import Computer Visionimport osimport sys, getoptimport signalimport timefrom edge_impulse_linux.image import ImageImpulseRunnerimport subprocess #this one have been added by Bera… elif "bounding_boxes" in res["result"].keys(): print('Found %d bounding boxes (%d ms.)' % (len(res["result"]["bounding_boxes"]), res['timing']['dsp'] + res['timing']['classification'])) if (len(res["result"]["bounding_boxes"])>0): exitCode = subprocess.call(["espeak","-ven+f3","-a200"," Found %d Buttons" % len(res["result"]["bounding_boxes"]) ]) #This one have been added by Bera…
…
Epseak is stand alone text-to-speech module for python. It does not require internet for workings.
Modified run:Now you have modified the python program. If you run the python file now, it will locate the button [on the top left, a small 120x120 camera port will open] and the numbers will be shown on the terminal window and the associated speaker will speak out the number – Found five buttons / Found 2 buttons etc. If you want to run some relay , light an LED etc. import the GPIO library of the python and then fire the associated GPIO to run the relay etc. However, for running a relay, you have to use a switching transistor to increase the amount of current required for running the relay! Aftermath: Edge impulse computingis started in 2019 with an objective to enable developers to create the next generation of intelligent devices. The AI powered programs / devices started appearing on ESP32, Jetson Nano , Raspberry Pi, Orange Pi, Maixduino, OpenMV , Nicla Vision and many more. This trend will further improve over the coming days! Gone are the days of super computers or big brand big sized computers. Small low powered modular devices will cover that space in the coming ahead.
Attachment: model.eim, linux-sdk-python, classify-image.py
Bye bye
S. Bera / Kolkata
Prelude : Classify of object is easily possible by edge impulse models. You can easily identify between a man & animals, between bi-cycle & other types of cars and so on. In the same coin you can easily count one type of object among other types of objects. This project at the beginning was aimed to install on MCU level – ESP32, Arduino Nicla vision etc. And that’s why it was made for very small area of counting [120 pixel x 120 pixel ] with relatively small size button as object of interest but finally it transpired that even for the smallest area too the MCUs are no match at all. The model file itself is about 8MB long! Therefore, the project was finally installed in a Raspberry Pi computer where it works easily.
Edge Impulse: First open an account in edgeimpulse.com which requires an email id. Collect similar types of buttons handful numbers. If you open the site from a Raspberry Pi computer, using the camera of the raspberry pi computer [either USB connected or cam port connected] you can collect images of buttons from several angles [which is required while the model is deployed in real working field]. However, edge impulse has provisions to connect your cell phone or laptop etc. to connect to for input device which is also more convenient for data acquisition in edge impulse project.
The project : The edge impulse project is broadly divided in the following steps –
1. Data acquisition – this could be image, sound, temperatures, distances etc. Part of these data are separated as test data while all other data are used as train data.2. Impulse design – this is sub divided into Create Impulse – sub divided by1. Input parameters – image [ width, heigh], sound [sound parameter]2. Processing block - How to process the input data3. Learning block - [object data of this model]
3. Image processing - Generate Feature of the collected images
5. Object detection – Select your Neural Network model and train the model.
In the final part – the object detection part needs your expertise or I would rather call it – trial and error effort so that the accuracy of the model becomes 85% or above. There are quite a handful models which you can try and see the accuracy level of the model. Anything above 90% is great but certainly it should not be 100% accurate! If it is so then you have something wrong in your data – could be very less data or insufficient features are there. Recheck & retry again for that case! For this project the accuracy was 98.6%. Certainly our number of data [about 40] was less. However, for a starter project this is pretty good! See the picture below.
Model Testing: You can test your model on the test data first. See how it works out there and then point your device to the real life data and see how it works! On the browser page look at this feature –
In the dashboard of the edge impulse opening page this feature is available. You can scan the image on your mobile and then run the model there or you can straight away run it in the browser. Point the camera to the buttons and see whether it can able to count them or not!
Raspberry Pi deployment : For running the model on Raspberry Pi computer you have to download the *.eim file on raspberry pi computer. But unlike for other hardware [Arduino, Nicla Vision, ESP32 where you can download directly] in case of Raspberry Pi you have to install edge impulse on the Raspberry Pi computer first. From inside that edge-impulse-daemon software you have to download this file. But don’t worry, edgeimpulse has devoted a full page for installing edgeimpulse on Raspberry Pi. Look at here – it’s pretty easy.
https://docs.edgeimpulse.com/docs/development-platforms/officially-supported-cpu-gpu-targets/raspberry-pi-4
OK, so you have installed edgempulse on the Raspberry Pi computer now. Now Run
edge-impulse-linux-runner [Remember to keep the Internet on for Raspberry Pi now]
on a Raspberry Pi computer terminal and see it connects to your edge impulse page [run edge-impulse-linux-runner --clean to switch projects]. This command will automatically compile and download the AI model of your project and then start running on your Raspberry Pi computer. [see command] Show the buttons to the camera connected to your Raspberry Pi and it would count them.
Deploy model in python: OK so far so good. In the above deployment, it would work as it is intended in the edge impulse model. To make it work for your special purpose , say raise an audio alarm / light an LED when the count reaches +2 you have to find some other means! Here comes python3 to help you out. Linux-sdk-python needs to be installed now in your Raspberry Pi computer.
Edge impulse SDK [Short form for Software Development Kit] is available for many models – Python, Node.js, C++ etc. The below link is for taking you to the page for SDK python page.
https://docs.edgeimpulse.com/docs/tools/edge-impulse-for-linux/linux-python-sdk
Once the Linux-SDK-Python is installed then go to the linux-sdk-python/examples/image directory and run the python file for image identification. Don’t get confused. In the example directory there are three sub directories – one each for audio data, image data and custom data. In the image directory the video classification file is also available for video input data. The custom directory is for customizing other kinds of data [ For experts area only!].
$> python3 classify-image.py /home/bera/downloads/model.eim
That’s how you have to load the python file with the downloaded model.eim file. The program will automatically find the camera module [USB connected or Cam-Port connected] and will start running! On the top left corner a small 120x120 camera window will open and the identified buttons will be market with small spot! The numbers identified will be shown on the terminal. Please ensure sufficient light is available and the camera is properly focused for the buttons. This is particularly important for cheap cameras. That’s why if you run the model on your smart phone it produces far superior images and counts far more quickly. Nonetheless ensure proper light and focus, it will also produce better result.
Customize your model: Please have a look into the classify-image.py file and it’s a not-a-simple python file which can be tailor made with little understanding. In this python file I’ve added espeak module such that the moment it finds button(s) ,it speaks out the number of button(s) it finds.
Lets see part of the python file to work around:-
#!/usr/bin/env pythonimport device_patches # Device Specific patches – taken care by the softwareimport cv2 #import Computer Visionimport osimport sys, getoptimport signalimport timefrom edge_impulse_linux.image import ImageImpulseRunnerimport subprocess #this one have been added by Bera… elif "bounding_boxes" in res["result"].keys(): print('Found %d bounding boxes (%d ms.)' % (len(res["result"]["bounding_boxes"]), res['timing']['dsp'] + res['timing']['classification'])) if (len(res["result"]["bounding_boxes"])>0): exitCode = subprocess.call(["espeak","-ven+f3","-a200"," Found %d Buttons" % len(res["result"]["bounding_boxes"]) ]) #This one have been added by Bera…
…
Epseak is stand alone text-to-speech module for python. It does not require internet for workings.
Modified run:Now you have modified the python program. If you run the python file now, it will locate the button [on the top left, a small 120x120 camera port will open] and the numbers will be shown on the terminal window and the associated speaker will speak out the number – Found five buttons / Found 2 buttons etc. If you want to run some relay , light an LED etc. import the GPIO library of the python and then fire the associated GPIO to run the relay etc. However, for running a relay, you have to use a switching transistor to increase the amount of current required for running the relay! Aftermath: Edge impulse computingis started in 2019 with an objective to enable developers to create the next generation of intelligent devices. The AI powered programs / devices started appearing on ESP32, Jetson Nano , Raspberry Pi, Orange Pi, Maixduino, OpenMV , Nicla Vision and many more. This trend will further improve over the coming days! Gone are the days of super computers or big brand big sized computers. Small low powered modular devices will cover that space in the coming ahead.
Attachment: model.eim, linux-sdk-python, classify-image.py
Bye bye
S. Bera / Kolkata
Diskussion (0 Kommentare)